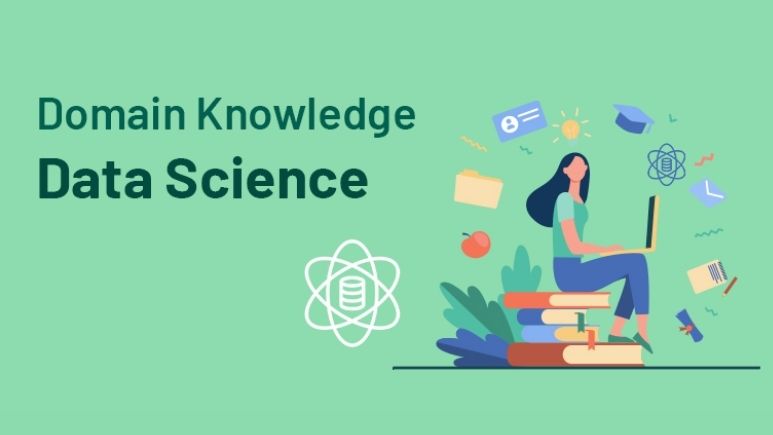
Data science is not just a field of computer science and mathematics, rather techniques or models that allow you to see the data in a purely scientific manner and use it in different ways. When working in an organization, not only are you processing data and finding patterns, but also preparing it for the companies to ask the right questions. It seems to be a small change for the company, but the data scientist has to apply a completely different approach for that.
This is the reason why you should know the ins and outs of data science and have domain knowledge. Moreover, the colleagues and clients asking questions about the data are from different backgrounds. They may not have the technical knowledge to understand complex patterns. In other words, they assume you have the domain knowledge and expertise to make sense out of the data and make them understand it.
If you’re a candidate with basic knowledge of programming and mathematics, or just know the concepts the lead to the foundation of data science, you can get the Data Science Training or Data Science Course and become an expert in this field. Now, let’s understand what domain knowledge means, its role in data science, and how it will help you get into the business.
Watch the video to get a better understanding of the data science concepts:
What is domain knowledge?
Simply put, domain knowledge refers to the understanding and information you have in a particular field. It describes your knowledge in that area which can be applied to solve specific problems, research, or a profession. In industries, domain knowledge determines your focus in the field and how well you create the workflows.
If you understand the domain and are able to connect or apply different technologies to drive meaningful solutions, you can easily face the challenges of that domain. Domain knowledge separates you from the candidates who’re only aware of the topics or technologies. To be an expert in a domain like data science, learn the core concepts, work on projects that challenge you in both technical and creative levels, learn new concepts from Data science tutorials, and get more certifications.
Role of Domain knowledge in Data Science
Domain knowledge of any field will take your work from the conceptual level to complex forms like predictive modeling, real-time analysis, mapping, and predicting future events. There are four main factors that one should consider when working on a data science project: they assess its quality.
- Precision
Precision is the level of uncertainty in your data values. Therefore it’s important for you to understand your industry and access those uncertainties for better results. There are different data Science tutorials that can help you find the right algorithms and techniques used to produce the desired results with high precision.
2. Accuracy
Similarly, accuracy determines the variation between your values and the default ones. It shows how well your project is performing and the consistency of your results.
3. Significance
Does your project address the nuances of the problem and genuine insights to already existing ones? If yes, then it has some significant value for both your company and domain. Otherwise, you need to put more effort in order to make it useful for the company and your team.
4. Representativeness
Do the datasets used are able to reflect all the characteristics of your domain? If not, then how much more datasets do you need to get the complete picture of it?
What Data Scientists should ask domain Experts
Following are the common questions that domain experts were asked by the Data Scientists:
- Your approach towards a problem statement?
Your approach determines your bias towards the problem statements or any situation. A purely scientific approach will mitigate the risks of you being biased and help you produce better results. By testing a specific hypothesis, your model will show the exact results expected by the data scientists.
2. What do you already know about?
It’s disappointing when you put down a lot of time and effort in making the project only to be known that it has redundancy and doesn’t add much knowledge to the domain. So, instead of spending time on what your colleagues and team members already know, ask the stakeholders about the feasibility of your project and follow a top-down approach to find the nuances that can bring some insights or positive changes in the domain.
3. What will you do with the results?
Before starting any project, your why should be clear about it, as it shows what you’ll do with the results. Drill down to individual detail, find what they’re looking for, and make a clear picture of what your results should look like. This will open the doors for new ideas and approaches you can take to solve the issue.
4. Is your model capable of producing accurate results?
Once you’ve built your model, it’s time to test it against varied parameters and find its limitations/strengths. If the model is underfitting then make changes to the model and test it again. Continue the process until you get satisfactory results.
Conclusions
So, that was all about domain knowledge, and I hope it helps you make models ready for the business world. The key to building successful models for a business is to become a linchpin. Meaning you have to understand the nuances of the problem and hold the complex structure of the models that benefit both your company and stakeholders.