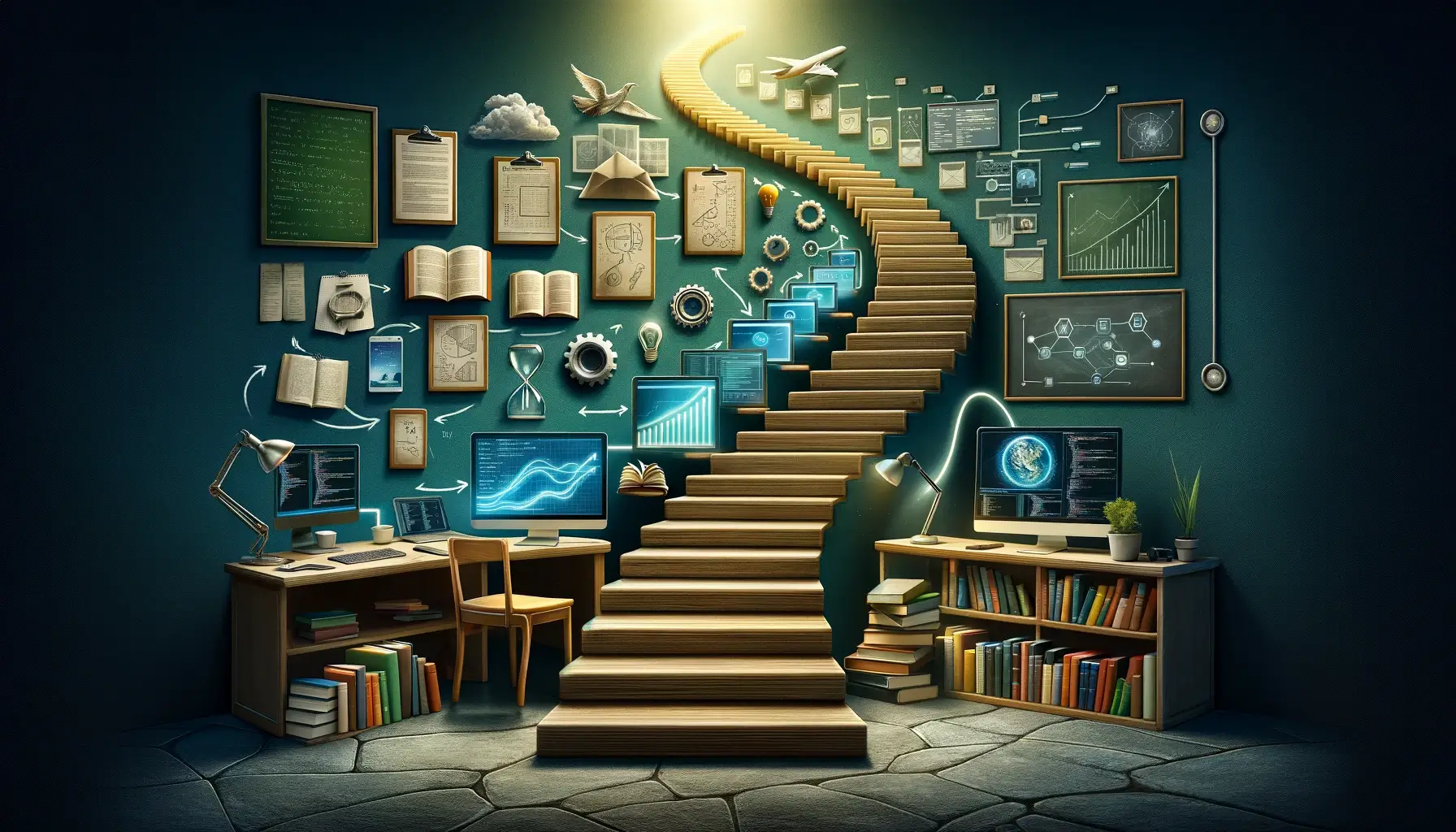
In the dynamic realm of data science education, the journey from a novice enthusiast to a proficient data scientist is marked by a structured progression pathway. Aspiring individuals keen on harnessing the power of data embark on this transformative voyage, seeking to navigate through the plethora of courses and resources available. Understanding the progression pathways in data science education is essential for charting a course towards professional proficiency and expertise.
The foundation of this journey often begins with enrolling in data science courses tailored for beginners. These introductory courses serve as gateways, providing learners with fundamental concepts, terminology, and tools essential for grasping the intricacies of data science. Here, individuals delve into subjects such as statistics, programming languages like Python or R, data visualization techniques, and basic machine learning algorithms. These foundational elements lay the groundwork for more advanced studies and practical applications.
As learners gain confidence and proficiency in the basics, they progress to intermediate-level data science courses. These courses delve deeper into specialized areas such as data manipulation, advanced statistical analysis, and the application of machine learning algorithms to real-world problems. Intermediate learners also explore data preprocessing techniques, model evaluation, and optimization strategies, honing their analytical skills and problem-solving abilities.
The transition from intermediate to advanced levels signifies a significant leap in expertise and specialization. Advanced data science courses offer in-depth exploration of complex topics such as deep learning, natural language processing, and big data analytics. Learners delve into advanced statistical modeling techniques, predictive analytics, and optimization algorithms, equipping themselves with the tools and knowledge required to tackle sophisticated data-driven challenges.
Become a data scientist entails not only theoretical knowledge but also practical experience. Hands-on projects and real-world case studies play a pivotal role in solidifying concepts and skills learned throughout the educational journey. These projects allow learners to apply theoretical knowledge to practical problems, analyze data sets, derive insights, and communicate findings effectively.
Furthermore, engaging in internships, apprenticeships, or participating in industry-sponsored projects provides invaluable real-world experience and exposure to the dynamics of the field. Collaborating with professionals in the industry offers opportunities for networking, mentorship, and gaining insights into the latest trends and best practices.
Continuous learning and professional development are paramount in the field of data science, given its ever-evolving nature. Beyond formal education, aspiring data scientists must stay abreast of emerging technologies, tools, and methodologies through self-study, online tutorials, workshops, and participation in conferences and seminars. Moreover, pursuing certifications and advanced degrees can further enhance one’s credentials and open doors to new career opportunities.
In conclusion, the progression pathway from beginner to pro in data science education is a multifaceted journey marked by continuous learning, practical experience, and professional development. By leveraging structured courses, hands-on projects, real-world experience, and ongoing self-improvement efforts, individuals can navigate this pathway with determination and perseverance, ultimately transforming themselves into proficient data scientists capable of making meaningful contributions to the ever-expanding field of data science.